The job market is on fire, which has many companies sweating over compensation. Is it competitive enough to effectively attract and retain talent? Early-stage startups are often reactive: grab some comp data to calibrate, but each offer is an individual discussion and decision. As companies scale, they start to ask: what’s our range for this role? We want to be in the top quarter of the market but what does that mean? For many organizations, the easiest path to answer these questions is market data from a compensation survey. These surveys collect data from multiple employers to determine the average compensation paid to employees in specific jobs.
While survey data can be a useful input, it’s important to balance your approach with other powerful data at your disposal.
The pitfalls of using raw compensation survey data
It can be so tempting to look at nicely formatted market data from a respected source like Option Impact or Radford and say “Let’s pay between 50th and 75th percentile of market. The numbers are right here, so we’ve got our pay ranges.” Be careful: an experienced comp analyst will tell you that data is messy.
So what are the 3 biggest pitfalls to watch out for with survey data?
Pitfall #1: The data is inconsistent. Consider how survey data is compiled - it’s aggregated and averaged across companies with different compensation approaches and philosophies. Can you imagine baking cookies by averaging the measurements across several recipes? Do you know if the recipes were aiming for chewy or crispy cookies? Would you trust that averaged recipe without more context?
The same holds for compensation data - take it with a grain (or pinch) of salt. Sometimes survey data seems illogical: e.g. a more senior level has a lower midpoint than a junior one; steps between levels might be too small, while others are too big.
Pitfall #2: The data is not aligned to your approach. If you’ve ever submitted survey data and struggled to choose job codes or thought “eesh, this one data point is an edge case”, you’re not alone. Survey data is aggregated across educated guesses from other companies trying to match their roles to job codes. And likely, these companies have a different interpretation and approach to compensation matters. Is their definition of a Senior Data Engineer the same as yours? Do they have the same philosophy on how that role should be paid? Is the compensation philosophy well defined and fully baked? Or malleable like cookie dough? Your company might be focused on the best chewy chocolate chip cookies while the survey reflects an assortment of cookies of all approaches and stages of development.
Pitfall #3: Survey data is a lagging indicator. Depending on the survey provider, all or some data may be 6 months old or more – stale data in a dynamic market like this. Also, compensation surveys measure what companies are currently paying existing employees. They don’t account for offers underway or compensation adjustments yet to take effect. Since many companies wait until a merit cycle to adjust existing employee comp for market changes, you may be seeing data that reflects comp ranges from a year ago or even longer.
Capture and develop your own data
So if market surveys are flawed, what can you do? Well, surveys have their place as one piece of the puzzle. Even if it’s your only dataset, data is only a starting point. It should ignite questions and thoughts about how compensation decisions will influence your company’s future (i.e., what’s fair compensation for existing employees and future candidates?).
As your company grows there are two additional datasets you can start collecting and developing internally. You likely already deal with both regularly, but may not have realized the value in treating them like datasets. Combined with market data, these three sources are core ingredients to informing compensation decisions.
Dataset #1: Internal compensation. What are the levels of your existing employees and how is their compensation distributed? Every company makes a series of comp decisions to grow and retain a team: compensation for your existing employees is a record of that. If you calculate the range and median for your employees at each level: it starts to look a lot like survey data and can be used to inform new comp decisions. If you decide to make changes for an individual offer or raise based on a comp survey or otherwise, you’ll come back to reconcile that change with how you’re paying other employees today. Internal compensation may be the most important data to consider, especially if your company supports equity and inclusion.
To take this thinking one step further, start calculating common compensation metrics like midpoint progression, salary range spreads, and salary range overlaps. If your company cares about career development and keeping employees engaged, these metrics will help inform a compelling compensation progression to match.
Dataset #2: Candidate offer data. Additionally, track the rich data that your recruiting team is building while making offers. Most recruiters ask candidates about compensation expectations and competing offers they should be aware of – don’t lose that data! Build a process or use a tool like Aeqium to capture market data points for actual candidates you’re trying to hire and use that as another perspective on how competitive your comp ranges are in the market. Also, track compensation for accepted and declined offers. Each of these data points is an opportunity to test your comp ranges in the market and learn how they’re received. You have much more context on these data points and can assess for relevant data vs outliers more easily than market survey data. (It also has the side benefit of helping your recruiters make more effective offers more quickly).
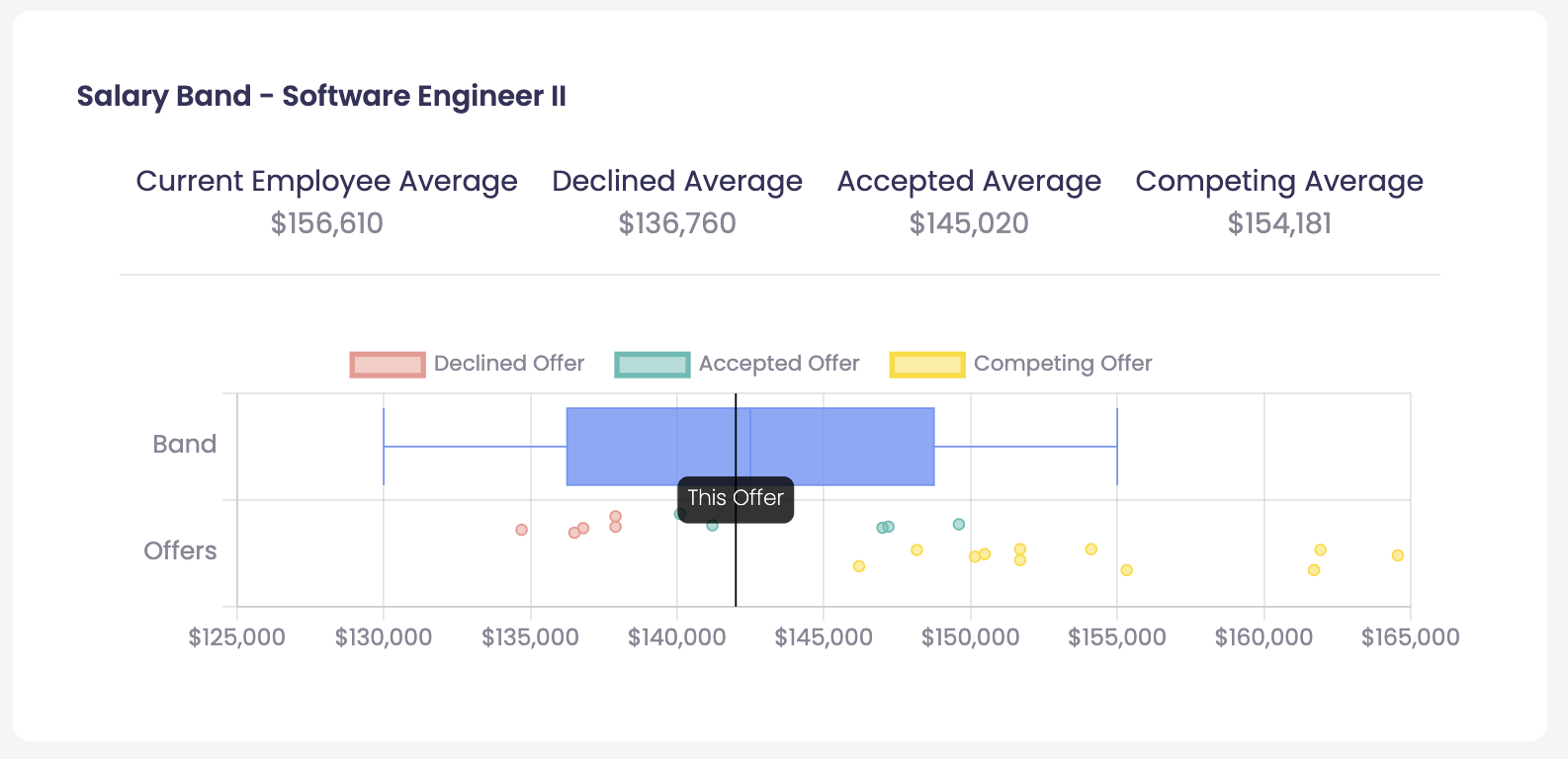
Triangulate across your data sources for better answers
Tracking these three datasets (market data, internal data, and candidate data) allows companies to make data-informed compensation decisions. Being intentional helps teams move faster and more proactively.
For example, a usual progression of events might be to extend market-based offers, see multiple offers declined due to compensation, and then react (e.g., work with the hiring manager based on internal data to determine how far to shift new hire offers).
In comparison, imagine you’re targeting to pay 50th percentile of market for a Level 5 role. The median of competing offers and expectations of recent candidates is 15% above the current median salary of your existing employees. That’s a good signal to consider a proactive market adjustment for current L5 employees. Add to the mix: data about departing employees who have left for higher compensation. You can further support this decision to adjust by looking at accepted and declined offers. If recent offers have been renegotiated or declined, that is a strong signal to recalibrate. For example, one option might be to look at the median across all candidate expectations and competing offers at that level for the last 6 weeks. Then adjust your band, and begin testing offers at up to 15% more than your previous range.
This approach works if you have existing employees and candidates to hire for a role. However, if you don’t yet have much data from candidate offers for a new role, survey data can be a useful starting point. Plan to learn and adjust quickly. For a new role, you can recalibrate your range even before you make your first offer. Pay special attention to competing offers and expectations from late-stage candidates: if they are meaningfully different from your survey data, pause to adjust your approach.
Consider how you’ll balance your candidate data with the ranges from your survey. Will you rely solely on survey data ranges given all the potential ways that it can be flawed? After reading this article, probably not! But at the same time, you will not blindly offer exactly what a single candidate is asking for. By looking at your datasets, you can navigate the decision with collective data and informed metrics.
Establishing your company’s offers or pay ranges directly from survey data might feel like the easiest approach to compensation. But, you might be missing critical ingredients in the recipe to compensation success. Instead, use all your data sources, with particular emphasis on data from existing employees or active candidates. The best part: Building a balanced, structured data foundation enables a more thoughtful outcome and it doesn’t have to take much more time.